Forecasting
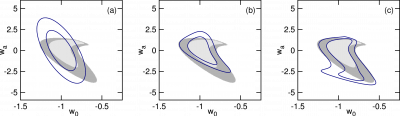
Theoretical physics poses questions about nature that have to be answered by taking data. However, building and conducting an experiment is a tedious and painstaking effort and often practical necessities enforce decisions about the design. Theoreticians can optimize these decisions by forecasting which experimental design will ultimately lead to the most constraining data.
Additionally, building the most precise experiment, doesn't automatically guarantee the most precise answers to the questions one has asked: The data also have to be analysed with a precision similar to that of the actual measurements. However, if the analysis shall be executable in less than a Hubble time, then idealizations are often mandatory. Forecasting tools are also helpful here: They can be used to asess into which analysis steps much computational effort should be investigated and when an idealizations is enough in order to make optimal use of the new data.
In our working groups, we test and extend the current standard model of cosmology, with the aim of finding detailed and satisfying answers to the problems of dark energy and dark matter. As such, we often predict hitherto unobserved phenomena that might appear if our universe is described by another model than the current LambdaCDM-cosmology. These new predictions will only be observable with future data – we therefore help to optimize experiments like the ESA-satellite Euclid.
Naturally, predicting new phenomena introduces new parameters to the model of our universe. These new parameters are often at best loosely constrained by current data – from a statistical point of view this means that their likelihoods are often decidedly non-gaussian. We therefore developed a new forecasting tool, DALI ( see arxiv:1401.6892 and image left), that is able to deal with non-Gaussian likelihoods. With this tool, we can safely go beyond the well sampled parameter space of the standard model and out into the sofar sparsely sampled parameter space of new physics – hopefully to convince observational astronomers to concentrate their efforts on these new spaces.